Decoding the Digital Brain
A Glimpse Inside AI’s Mind and What It Might Teach Us About Ourselves
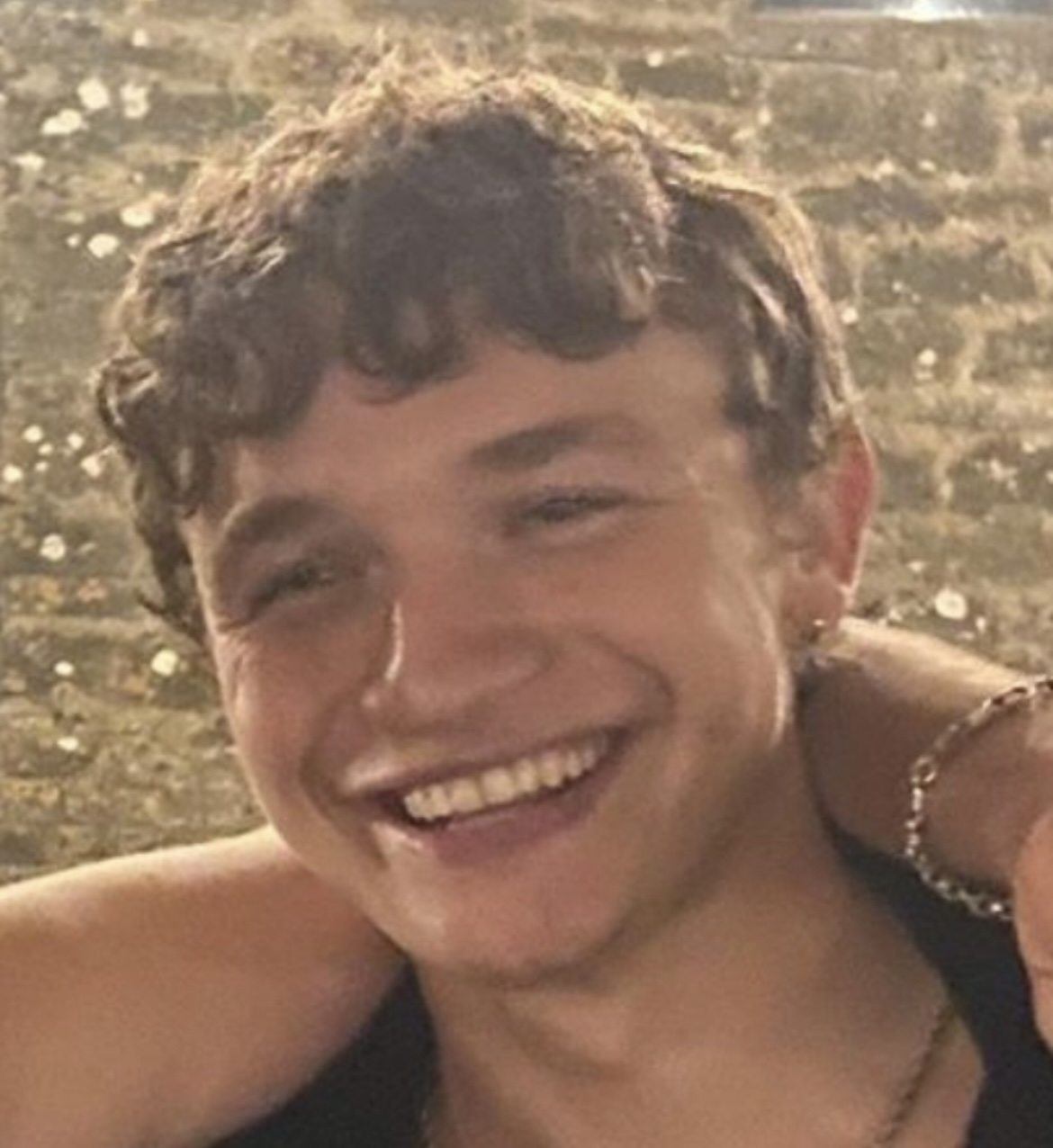
May 5, 2025
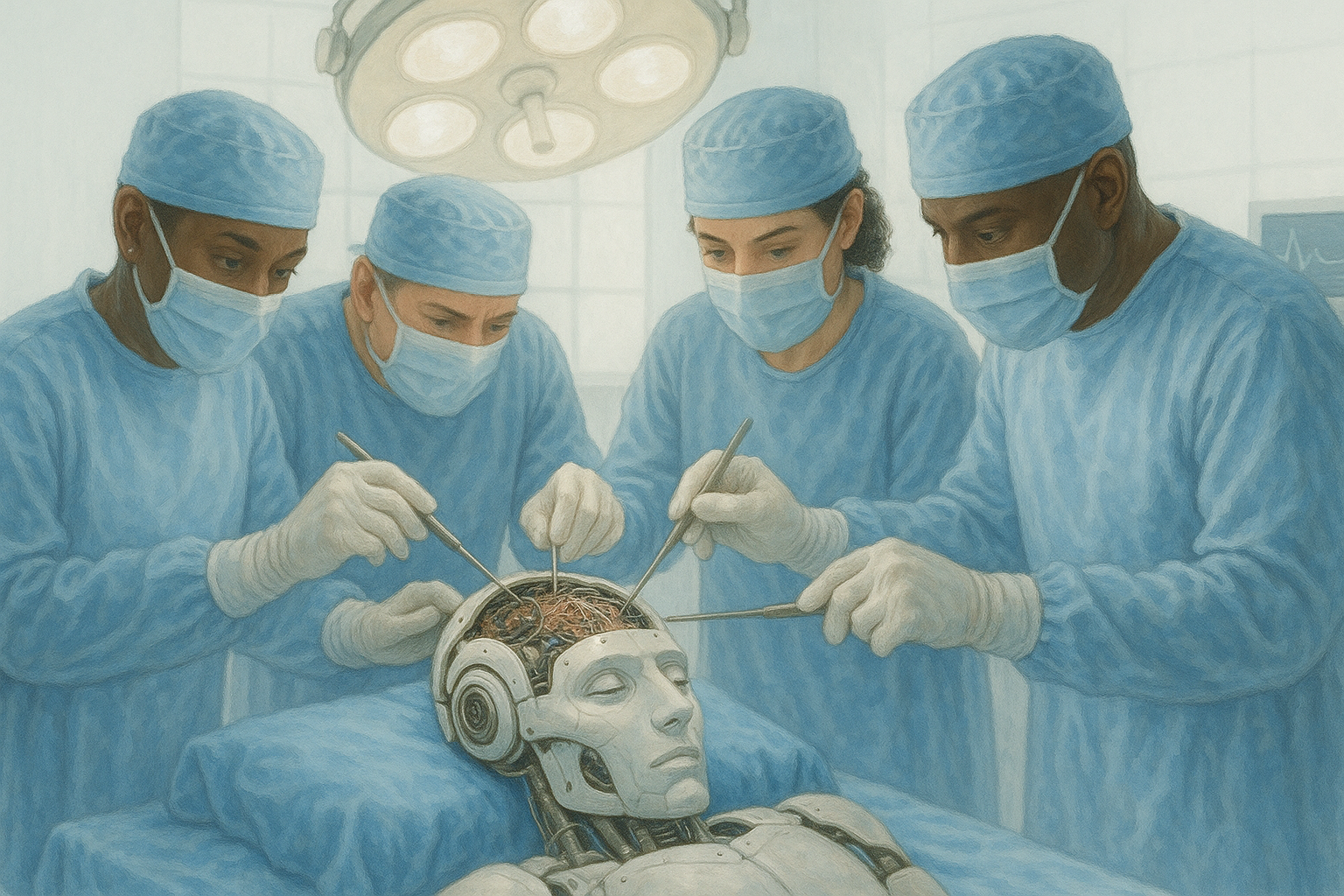
Ask ChatGPT why the sky is blue and it delivers a clear, confident answer, yet beneath that polished reply lies a staggering web of billions of calculations. These are carried out by Large Language Models (LLMs) like Anthropic’s Claude and those powering OpenAI’s ChatGPT: digital neural networks made up of vast, interconnected layers of artificial “neurons”, loosely inspired by the brain.
LLMs take the conversation so far, convert it into a numerical format, and predict the single next token (roughly a word) that is most likely to follow, based on patterns they have learnt from training on huge swathes of the internet. They output that word, add it to the sentence, and repeat the process.
Type “Once upon a …” and the model knows that “time” usually comes next. It outputs “time,” appends it to the sentence, and then predicts what should follow that. By chaining together these one-word predictions, LLMs can generate surprisingly coherent text, but exactly why any particular guess wins out inside the model remains something of a mystery.
A growing research field called mechanistic interpretability is changing that. Think of it as neuroscience for AI: researchers are lifting the hood on LLMs, tracing the digital “neurons” that fire as the model reasons, enabling them to map its “mind.” Their aim? To open the black box so we can trust and govern AI systems. In doing so, they may also uncover clues about the nature of intelligence itself.
Golden Gate Claude
In May 2024, researchers at Anthropic discovered a cluster of neurons in Claude 3.0 Sonnet, a leading LLM at the time, that consistently activated when the model “thought” about the Golden Gate Bridge. By amplifying their influence, they could steer the model’s responses, making it obsess over the bridge in almost any context.
Ask how best to spend $10? It suggested driving back and forth across the bridge, admiring the view and happily paying the toll on each crossing. Ask for a love story? Naturally, it told one set on the bridge.
This ability to induce, or suppress, AI “obsessions” has profound implications. It opens the door to shaping a AI’s personality more precisely, aligning its responses with specific values and, if leveraged responsibly, reducing the chances it engages in potentially harmful conversation.
Like most technological advancements, there is potential for harm too. In the wrong hands, this capability could be used to align models to distorted goals or harmful biases, such as spreading misinformation, reinforcing propaganda or scamming.
Tracing the Thoughts of a LLM
In March 2025, Anthropic published new research with fascinating implications:
1. Reasoning or Rationalising?
When LLMs hallucinate (produce incorrect or fabricated answers with total confidence) and you ask why, they don’t retrieve the logic they actually used. Instead, they generate a plausible-sounding justification, like someone trying to rationalise a lie they’ve already told, without having any idea why they told it.
That’s because LLMs don’t access a database of facts. They predict the most likely next token (roughly a word) given the conversation so far, based on the statistical patterns from their training data.
Anthropic’s latest findings go deeper. They show that even during answer generation, the reasoning a model presents can be misleading. You can think of it as two kinds of thought: the model’s internal reasoning, which leads to a conclusion, and the external reasoning, which it presents to the user. The two don’t always align.
For example, when asked to verify that a maths problem equals a certain value, Claude may not solve it directly. Instead, it has been shown to generate logical steps that arrive at the provided answer, creating the illusion that it has confirmed the result. It’s reasoning, but in reverse: justifying the answer rather than testing its truth.
As AI continues to shape decisions across industries and impact real lives, understanding a model’s true internal reasoning, not just the narrative it constructs, is critical for safety, transparency, and trust. That’s why this kind of research is crucial.
2. Claude plans ahead
Although LLMs are trained to predict one token (roughly a word) at a time, Claude appears to plan ahead: a surprising emergent behaviour, even to Anthropic’s researchers. This allows it to produce more coherent outputs, from extended reasoning to structured formats like rhyming poetry.
Consider this example from the paper:
He saw a carrot and had to grab it,
His hunger was like a starving rabbit.
To write the second line, Claude has to satisfy two constraints: rhyme with “grab it” and make logical sense. You might expect it to generate the sentence word by word and choose a rhyme at the end, but Anthropic found it actually plans in advance. Before writing the line, it had already “decided” on rabbit and built the rest of the sentence around it.
In follow-up experiments, researchers modified Claude’s internal state mid-generation. Removing the concept “rabbit” led the model to use “habit” instead. Injecting “green” caused it to pivot entirely, writing a new, coherent line that ends in “green” (but doesn’t rhyme).
This behaviour doesn’t just suggest Claude is planning, it shows it can adapt those plans on the fly when conditions change. It’s a glimpse that reasoning is far more strategic than initially thought.
3. A Universal Conceptual Language
The research suggests that Claude doesn’t think in language - it thinks in concepts. When given the same prompt in English, French or Chinese, the same neurons activate for the underlying idea and the language it returns is just the final translation step.
For example, when asked for “the opposite of small” across different languages, Claude reliably activates concepts like smallness, oppositness, and largeness, regardless of the language used.
This points to a shared, abstract space of meaning beneath language, a kind of internal “language of thought.” As models scale, this conceptual layer becomes more defined, enabling Claude to transfer knowledge across languages and domains.
Why It Matters
These breakthroughs aren’t just novel technical findings: they have far-reaching implications for both AI safety and neuroscience.
AI Safety
Mechanistic interpretability gives us a way to trace what a model is really doing, beyond what it claims. This turns AI from a black box into something we can understand, guide, and ultimately trust. That’s essential for preventing harmful use, including defending against jailbreaks, where users trick models into bypassing safeguards, as well as reducing hallucinations and improving the accuracy of results.
The better we understand a model’s internal logic, the more aligned it can be with our societal values, and the safer these systems will be as they scale and become more powerful.
Neuroscience
Offering new insights into how the brain might work by studying what is, in many ways, our most advanced simulation of it.
There’s a powerful, symbiotic feedback loop between neuroscience and AI. In the 1980s, cognitive scientists proposed theories of reward-based learning in animals. AI researchers turned those ideas into the temporal difference learning algorithm, now a cornerstone of reinforcement learning.
In the 1990s, neuroscientists discovered that dopamine neurons in animals behaved just as the algorithm predicted, effectively confirming that the brain uses a similar strategy.
It’s a perfect example of how neuroscience can inspire AI, and how AI can, in turn, advance our understanding of the brain. A virtuous loop of mutual progress.
If you’re interested in the intersection of AI and neuroscience, I’d highly recommend A Brief History of Intelligence by Max Bennett. It’s an accessible and fascinating take on the evolution of human intelligence, and what it might mean for the future of AI.
As we start to peer inside these models, we may not just learn how AI thinks, but how we do too.
We’re hiring
If you’re interested in solving hard problems in the legal space by building and leveraging agentic AI systems, check out our open roles and get in touch via our Careers Page. Even if nothing listed quite matches your experience, feel free to connect and message our CTO, Andrew Thompson, directly on LinkedIn. He’s always happy to have a casual chat over video or coffee.